Optimizing LLM Outputs with Dual-Perspective Prompt Engineering
Discover how hybrid prompt engineering, combining expert analysis with audience-focused communication, produces superior LLM outputs for business leaders.
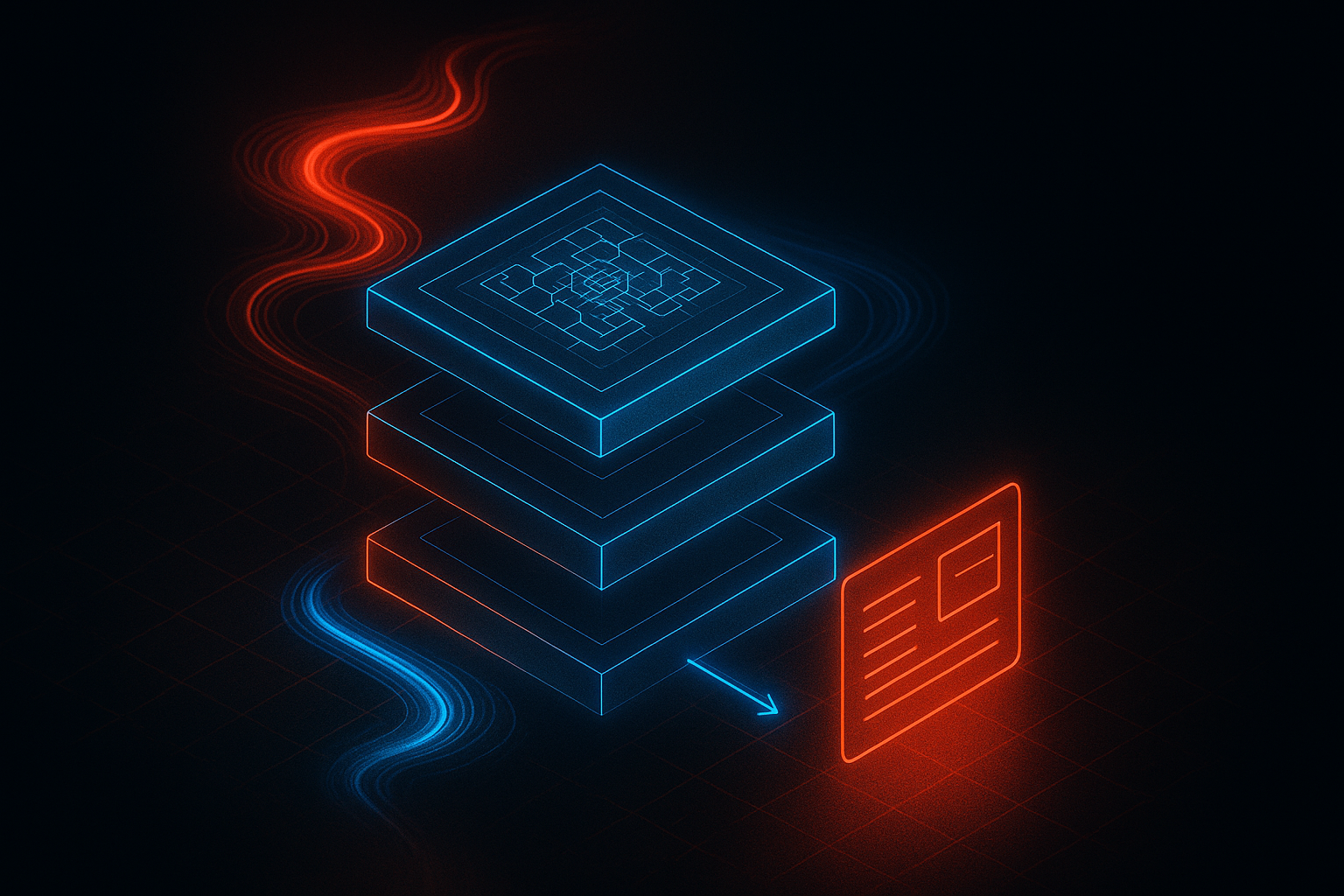
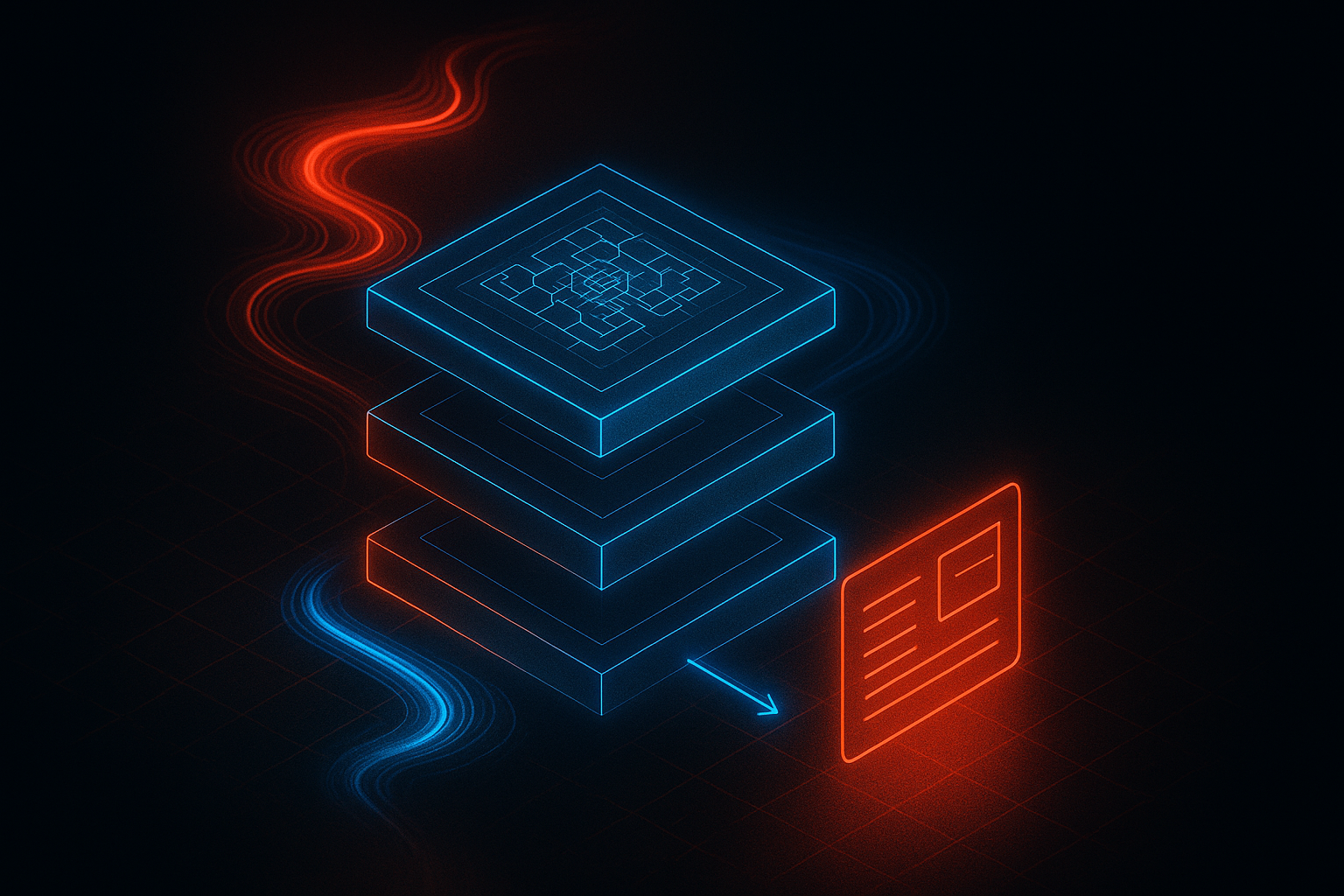
We’ve recently partnered with a client to build an internal AI-powered interface that delivers actionable insights to different personas (e.g., executives, team leads, and ICs). Rather than a typical chatbot, our interface surfaces curated insights based on pre-generated prompts that are connected to realtime data sources. During the prompt engineering process, we asked ourselves a foundational question:
What makes a prompt good enough to generate truly useful insight, not just a plausible answer?
More specifically, should our prompts be designed from the perspective of the persona receiving the insight, or from the persona best suited to generate it? A tension reflected in prompt engineering as “Explain ABC to a CMO” vs. “Act as a data analyst analyzing XYZ.”
The Hypothesis
Our working theory was that emphasizing the persona best suited to generate the insight would produce higher-quality, more actionable outputs than prompts written from the POV of the intended recipient. We suspected that prompting from the audience’s POV might constrain the model to overly simplistic, surface-level answers that would never lead to real actions and outcomes.
However, our goal here was a truly useful insight, and the former approach could lead to expert-level outputs that are impressive, but unusable in practice. Thus we wanted to test whether prompting the model to first reason as an expert, then communicate to a specific persona (mimicking natural communication flow), would generate richer and more useful insights.
Designing the Experiment
We needed a real-world scenario to test and picked a contemporary challenge that will feel familiar to folks in the financial services (FSI) space: The impact of generative AI on retail banking including risks, opportunities, and implementation strategies. With the topic selected, we then created our three prompt styles:
Prompt A: Expert POV
“Act as an AI strategy consultant with experience in digital transformation. Analyze the impact of generative AI on retail banking.”
Prompt B: Audience POV
“Explain to a Senior VP of Retail Banking how generative AI could affect their business in the next 1–2 years.”
Prompt C: Dual POV
“Act as an AI strategy consultant… Then translate your analysis into a briefing for a Senior VP of Retail Banking that will inspire them to take action with clarity and confidence.”
We evaluated the outputs on a few key dimensions including clarity, depth, actionability, and audience alignment.
Comparative Results*
*Prompts tested across Gemini Advanced 2.0 Flash, Claude Sonnet 3.7, and ChatGPT 4o Pro
Clarity
Prompt A (Expert POV)
- Used academic language and complex sentence structures
- Information was organized systematically but densely
- Numbered references created a scholarly tone but interrupted flow
- More difficult for non-experts to quickly process
Prompt B (Audience POV)
- Used simpler, more accessible language
- Employed clear categorization and shorter paragraphs
- Included concrete examples to illustrate points
- Prioritized readability over comprehensiveness
Prompt C (Dual POV)
- Employed direct, confident language with strategic framing
- Used a compelling narrative structure with clear sections
- Balanced technical content with accessible explanations
- Formatted information in digestible chunks
Winner: Prompt C (Dual POV): It maintained clarity while conveying sophisticated concepts, using executive-appropriate language.
Depth of Analysis
Prompt A (Expert POV)
- Provided comprehensive coverage of generative AI applications
- Included detailed explanations of technical concepts
- Contained extensive supporting examples
- Addressed multiple dimensions (technical, operational, ethical)
Prompt B (Audience POV)
- Focused on business implications over technical details
- Simplified complex concepts, sometimes at the expense of nuance
- Emphasized practical applications over theoretical possibilities
- More selective about which topics to cover deeply
Prompt C (Dual POV)
- Maintained depth on strategically important topics
- Incorporated specific metrics and quantitative insights
- Included both immediate and long-term strategic considerations
- Balanced technical depth with business relevance
Winner: Prompt A (Expert POV) for pure depth, but Prompt C (Dual POV) for abalance that remained relevant to the audience.
Actionability
Prompt A (Expert POV)
- Contained recommendations but they're often abstract
- Lacked specific implementation guidance
- Didn't prioritize actions by impact or feasibility
- More focused on explaining than guiding action
Prompt B (Audience POV)
- Included more practical implementation suggestions
- Focused on near-term opportunities (1-2 year timeframe)
- Sometimes lacked the strategic context for prioritization
- More concrete but sometimes lacked the "why" behind recommendations
Prompt C (Dual POV)
- Provided specific, prioritized action steps
- Included implementation timelines and resource considerations
- Connected actions to strategic outcomes
- Offered concrete next steps
Winner: Prompt C (Dual POV): It provided the most specific and actionable guidance while maintaining strategic context.
Audience Alignment
Prompt A (Expert POV)
- Written for peers or academic audiences
- Assumed significant background knowledge
- Focused on completeness over relevance to decision-makers
- Didn't address executive priorities specifically
Prompt B (Audience POV)
- Written with executive perspective in mind
- Focused on business outcomes and competitive positioning
- Addressed common executive concerns (ROI, timeframes, risks)
- Sometimes oversimplified for the sake of accessibility
Prompt C (Hybrid)
- Perfectly calibrated for senior executive audience
- Addressed both strategic and operational concerns
- Spoke to leadership challenges and competitive positioning
- Used executive-appropriate framing and language
Winner: Prompt C (Dual POV): It demonstrated the strongest understanding of the executive audience's needs and perspectives.
What We Learned
Great prompt design mirrors human communication and collaboration. We don't just think deeply; we adapt how we deliver our thinking depending on who we're talking to.
In the experiment, the hybrid prompt consistently outperformed the others across nearly every key measure. By explicitly instructing the AI to both analyze as an expert and then translate for a specific audience, it leveraged the strengths of both approaches while minimizing their limitations. This led to more insightful and usable responses that a leader could confidently share with their team and drive action.
As a Repeatable Framework
If you're building prompts for internal tools, knowledge delivery, or AI copilots, try this two-step structure:
Think as the expert: Prompt the AI to analyze or explore from the point of view of someone with relevant domain knowledge.
→ "Act as a compliance officer, evaluate X..."
Speak to the audience: Then reframe that output so it’s clear and useful for the intended stakeholder.
→ "...Now summarize your findings for a business unit lead."
You can design your system to automate this two-step reasoning, first prompting for the expert output, then auto-chaining a second prompt for the audience translation.
Final Thoughts
While much of the social discourse around prompt engineering emphasizes clever hacks, persona tokens, or verbosity tricks; the reality of good prompt engineering is much simpler: it’s about aligning your prompt with how people naturally think, communicate and collaborate in your organization.